Acquiring musculoskeletal skills using AI
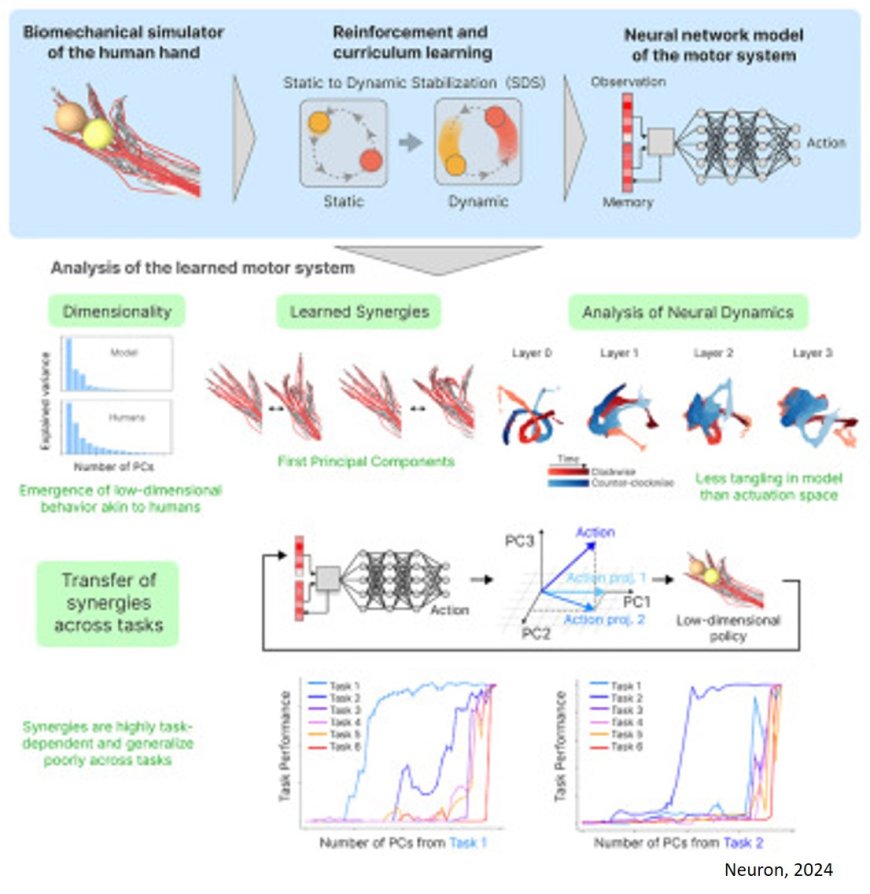
In neuroscience and biomedical engineering, accurately modeling the complex movements of the human hand has long been a significant challenge. Current models often struggle to capture the intricate interplay between the brain's motor commands and the physical actions of muscles and tendons. This gap not only hinders scientific progress but also limits the development of effective neuroprosthetics aimed at restoring hand function for those with limb loss or paralysis.
The team have developed an AI-driven approach that significantly advances our understanding of these complex motor functions. The team used a creative machine learning strategy that combined curriculum-based reinforcement learning with detailed biomechanical simulations.
The NeurIPS challenge by Meta motivated the team to find a new approach to a technique in AI known as reinforcement learning. The task was to build an AI that precisely manipulate two Baoding balls—each controlled by 39 muscles in a highly coordinated manner. This seemingly simple task is extraordinarily difficult to replicate virtually, given the complex dynamics of hand movements, including muscle synchronization and balance maintenance.
In this highly competitive environment, three graduate students outperformed their rivals by a significant margin. Their AI model achieved a 100% success rate in the first phase of the competition, surpassing the closest competitor. Even in the more challenging second phase, their model showed its strength in ever more difficult situations and maintained a commanding lead to win the competition.
“To overcome the limitations of current machine learning models, we applied a method called curriculum learning. After 32 stages and nearly 400 hours of training, we successfully trained a neural network to accurately control a realistic model of the human hand,” says one of the authors.
A key reason for the model’s success is its ability to recognize and use basic, repeatable movement patterns, known as motor primitives. In an exciting scientific twist, this approach to learning behavior could inform neuroscience about the brain’s role is in determining how motor primitives are learned to master new tasks. This intricate interplay between the brain and muscle manipulation points to how challenging it can be to build machines and prosthetics that truly mimic human movement.
“You need a large degree of movement and a model that resembles a human brain to accomplish a variety of everyday tasks. Even if each task can be broken down into smaller parts, each task needs a different set of these motor primitives to be done well,” says the senior author.